How to Use Historical Data to Forecast Future Trends
In today's fast-paced world, the ability to predict future trends is not just a luxury but a necessity for businesses and organizations. Historical data acts as a crystal ball, offering insights that help in making informed decisions. By examining past events, patterns, and behaviors, organizations can gain a deeper understanding of what lies ahead. But how exactly do we harness this treasure trove of information? Let’s dive into the significance of historical data and how it can be effectively utilized to forecast future trends.
Understanding why historical data is crucial for forecasting helps organizations make informed decisions. It provides context and benchmarks that guide future predictions and strategic planning. Think of historical data as a roadmap; it shows you where you've been, which can help you navigate where you're going. Without this roadmap, you might find yourself lost in a maze of uncertainty. For instance, if a retail company analyzes sales data from previous years, it can identify peak shopping seasons and adjust inventory accordingly. This not only enhances efficiency but also boosts customer satisfaction.
Various methods exist for analyzing historical data, including statistical analysis, time series analysis, and regression models. Each method offers unique insights and can be tailored to specific forecasting needs. By employing the right analytical techniques, businesses can uncover hidden trends and make predictions that are not merely educated guesses but data-driven forecasts. Here’s a closer look at some of these methods:
Statistical techniques such as mean, median, and standard deviation help summarize historical data. These foundational analyses enable forecasters to identify trends and anomalies effectively. For example, understanding the average sales figures can help a business determine whether a particular product is performing well or if it’s time to pivot.
Descriptive statistics provide a summary of historical data, allowing forecasters to understand trends at a glance. This information is essential for making initial assessments of data patterns. By presenting data in a clear and concise manner, descriptive statistics can illuminate trends that might otherwise go unnoticed.
On the flip side, inferential statistics allow forecasters to make predictions about future trends based on sample data. This approach helps in drawing conclusions and making informed decisions despite incomplete information. Imagine you're trying to predict the outcome of a sports game; you could analyze past performance data to make a logical guess about future results.
Time series analysis focuses on data points collected or recorded at specific time intervals. It helps identify seasonal trends, cyclical patterns, and long-term movements in data. For example, a company might analyze its sales data over several years to pinpoint seasonal spikes, allowing them to prepare marketing strategies tailored to those peak periods.
Numerous tools are available for analyzing historical data and forecasting future trends, ranging from simple spreadsheets to sophisticated software solutions. Choosing the right tool can enhance accuracy and efficiency. Just as a chef chooses the best knives for cooking, businesses must select the right forecasting tools to slice through the data effectively.
Excel remains a popular choice for many businesses due to its accessibility and functionality. It offers various built-in functions and templates for basic forecasting needs. With features like pivot tables and charts, users can visualize data trends easily, making Excel a handy tool for quick analyses.
For those with more complex data needs, advanced forecasting software provides more robust analytics capabilities, including machine learning algorithms and data visualization tools. These solutions are ideal for organizations looking to leverage big data for predictive analytics. Imagine having a powerful engine that not only analyzes historical data but also learns from it to improve future predictions!
- What is historical data? Historical data refers to past information, records, or statistics that can be analyzed to identify trends and patterns.
- Why is historical data important for forecasting? It provides context and benchmarks, allowing organizations to make informed predictions about the future.
- What methods can be used to analyze historical data? Common methods include statistical analysis, time series analysis, and regression models.
- What tools are available for forecasting? Tools range from basic applications like Excel to advanced software solutions with machine learning capabilities.
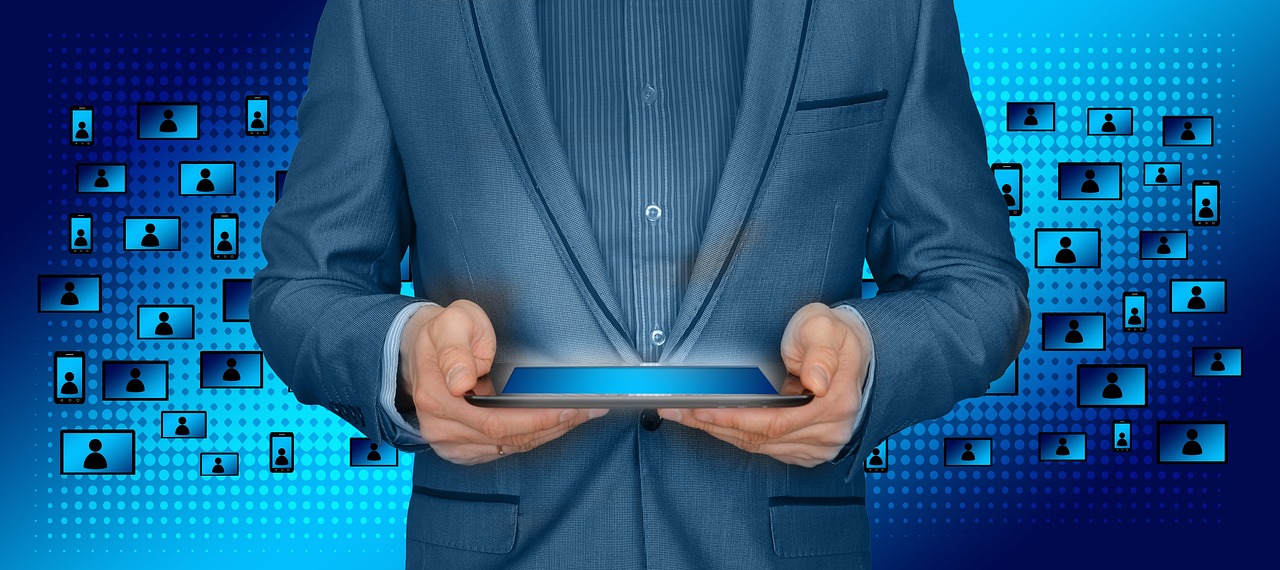
The Importance of Historical Data
Understanding why historical data is crucial for forecasting helps organizations make informed decisions. Imagine trying to navigate a ship without a map; that’s what forecasting without historical data feels like. Historical data provides context and benchmarks that guide future predictions and strategic planning. By analyzing past trends, businesses can identify patterns that may repeat, allowing them to anticipate future challenges and opportunities.
When we talk about historical data, we're referring to the wealth of information collected over time. This data can include sales figures, customer behavior, market trends, and even economic indicators. Each data point acts like a puzzle piece, contributing to a larger picture that helps organizations visualize their trajectory. The ability to look back at this information allows companies to make decisions that are not just reactive but also proactive.
Furthermore, historical data is essential for risk management. By understanding past failures and successes, organizations can develop strategies to mitigate risks in the future. For instance, if a company notices a seasonal dip in sales every winter, they can prepare by ramping up marketing efforts in advance or adjusting inventory levels accordingly. This kind of strategic foresight is invaluable in maintaining a competitive edge.
Additionally, historical data aids in setting realistic goals. When organizations have a clear understanding of their past performance, they can set achievable targets based on actual trends rather than guesswork. This data-driven approach fosters accountability and can motivate teams to strive for continuous improvement.
To illustrate the significance of historical data, consider the following table that outlines key benefits:
Benefit | Description |
---|---|
Informed Decision-Making | Provides a foundation for making strategic choices based on past performance. |
Risk Management | Helps identify potential risks and develop strategies to mitigate them. |
Goal Setting | Enables organizations to set realistic and achievable targets. |
Trend Identification | Allows for the recognition of patterns that can inform future strategies. |
In summary, the importance of historical data in forecasting cannot be overstated. It's the backbone of strategic planning and operational efficiency. Organizations that leverage historical data not only enhance their predictive accuracy but also create a culture of continuous improvement. By understanding the past, they pave the way for a more informed future.
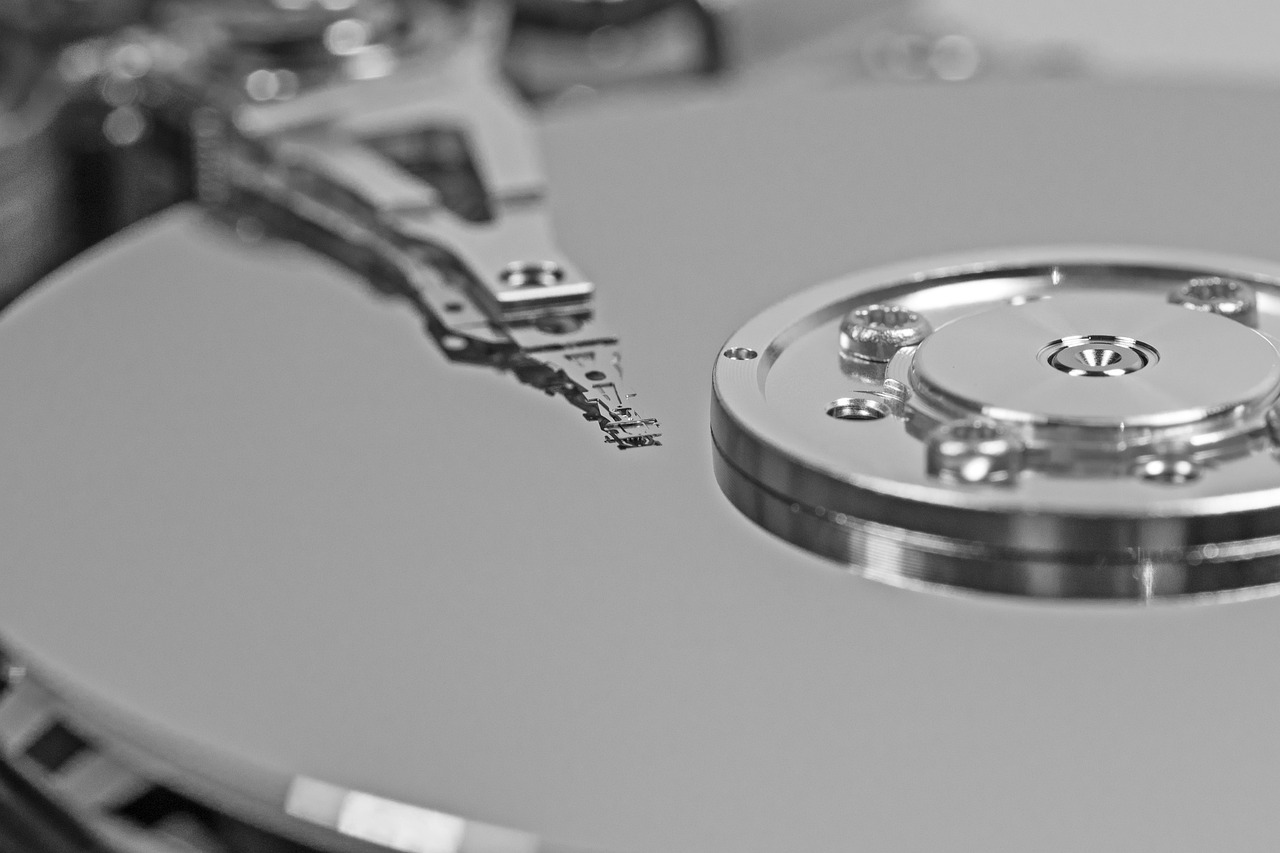
Methods of Analyzing Historical Data
When it comes to predicting future trends, the way we analyze historical data can make all the difference. Various methods exist, each offering unique insights that can help organizations tailor their forecasting strategies to meet specific needs. Think of it like choosing the right tool for a job; using the wrong one can lead to poor outcomes. So, let’s dive into the most effective methods for analyzing historical data!
One of the most fundamental approaches is statistical analysis. This method encompasses a range of techniques that summarize historical data, making it easier to identify patterns and trends. For instance, basic statistical measures like the mean, median, and standard deviation can provide a snapshot of the data's behavior. These foundational analyses allow forecasters to pinpoint anomalies that could indicate shifts in trends. Imagine you're looking at a stock's performance; understanding its average price over time helps you gauge whether it's on an upward or downward trajectory.
Next up is time series analysis, which focuses on data points collected at specific intervals. This method is particularly useful for identifying seasonal trends or cyclical patterns. For example, a retail company might analyze sales data over several years to determine how holiday seasons affect purchasing behavior. By recognizing these patterns, they can better prepare their inventory and marketing strategies. Time series analysis can be likened to watching the tides; by understanding past movements, you can predict when the next high or low tide will occur.
In addition to these methods, regression models can be employed to explore relationships between different variables. This technique allows forecasters to understand how various factors influence one another. For instance, if a company wants to know how advertising spending impacts sales, regression analysis can provide clarity. By examining historical data, businesses can make informed predictions about how changes in one area may affect another, much like how adjusting the temperature in an oven affects the outcome of a baked dish.
To summarize, here are the key methods of analyzing historical data:
- Statistical Analysis: Summarizes data to identify trends.
- Time Series Analysis: Focuses on data collected over time to spot seasonal trends.
- Regression Models: Examines relationships between variables to make informed predictions.
Each of these methods can be tailored to fit specific forecasting needs, allowing organizations to make informed decisions based on historical insights. By understanding the strengths and weaknesses of each approach, businesses can enhance their forecasting accuracy and develop strategies that are not just reactive but proactive. So, the next time you’re faced with a decision that relies on forecasting, remember the power of historical data and the methods at your disposal!
1. What is the best method for analyzing historical data?
The best method depends on your specific needs. Statistical analysis is great for summarizing data, while time series analysis is ideal for identifying trends over time. Regression models are useful for understanding relationships between variables.
2. How can I access historical data for analysis?
Historical data can often be found through public databases, industry reports, or your organization's internal records. Websites like government databases or financial market platforms can also be valuable resources.
3. What tools can I use for forecasting?
There are many tools available, from simple spreadsheet software like Excel to advanced analytics platforms that utilize machine learning algorithms. The choice depends on the complexity of your data and your specific forecasting needs.
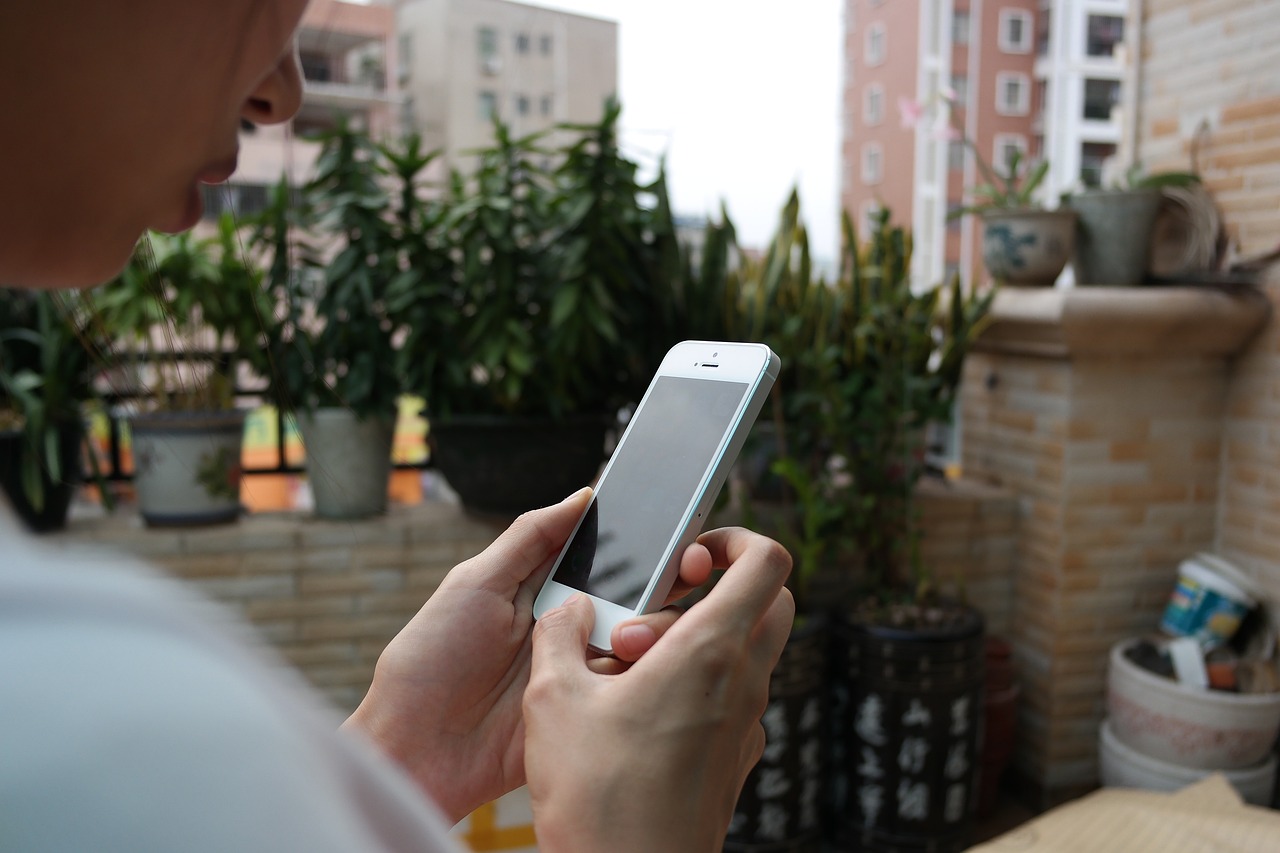
Statistical Analysis Techniques
When it comes to forecasting future trends, play a pivotal role. They allow businesses and researchers to sift through historical data and extract meaningful insights that can guide decision-making. Imagine you’re trying to find a needle in a haystack; statistical methods are your metal detector, helping you pinpoint that needle with precision.
One of the foundational techniques in statistical analysis is the calculation of mean, median, and standard deviation. These metrics provide a snapshot of your data's central tendency and variability. For instance, the mean gives you the average value, while the median indicates the middle point of your data set, which can be particularly useful in identifying outliers. The standard deviation measures the extent of variation or dispersion of a set of values, helping forecasters understand how spread out the data points are.
To illustrate, consider the following table that summarizes a fictional dataset on monthly sales over a year:
Month | Sales ($) |
---|---|
January | 5000 |
February | 6000 |
March | 7000 |
April | 8000 |
May | 7500 |
June | 9000 |
July | 9500 |
August | 10000 |
September | 11000 |
October | 12000 |
November | 13000 |
December | 14000 |
Using this data, a forecaster can calculate the mean sales per month, which would be a valuable indicator of overall performance. Additionally, understanding the standard deviation can help identify months that performed significantly better or worse than the average, allowing businesses to investigate the causes behind these fluctuations.
Another crucial aspect of statistical analysis is inferential statistics. This technique allows forecasters to make predictions about future trends based on a sample of data rather than the entire dataset. It’s like trying to predict the weather based on a few days of temperature readings; while it may not be perfectly accurate, it provides a reasonable estimate. By using inferential statistics, businesses can draw conclusions about their entire market based on the behavior of a smaller, representative sample.
In summary, statistical analysis techniques are essential for anyone looking to forecast future trends effectively. They not only help summarize historical data but also enable forecasters to make informed predictions that can shape strategic decisions. By leveraging the power of these techniques, organizations can navigate the uncertainties of the future with greater confidence.
- What is the primary purpose of statistical analysis in forecasting? Statistical analysis helps summarize historical data and extract insights that guide future predictions.
- How do mean, median, and standard deviation differ? The mean is the average, the median is the middle value, and the standard deviation measures data variability.
- Why are inferential statistics important? They allow predictions about a larger population based on a smaller sample, making forecasting more efficient.
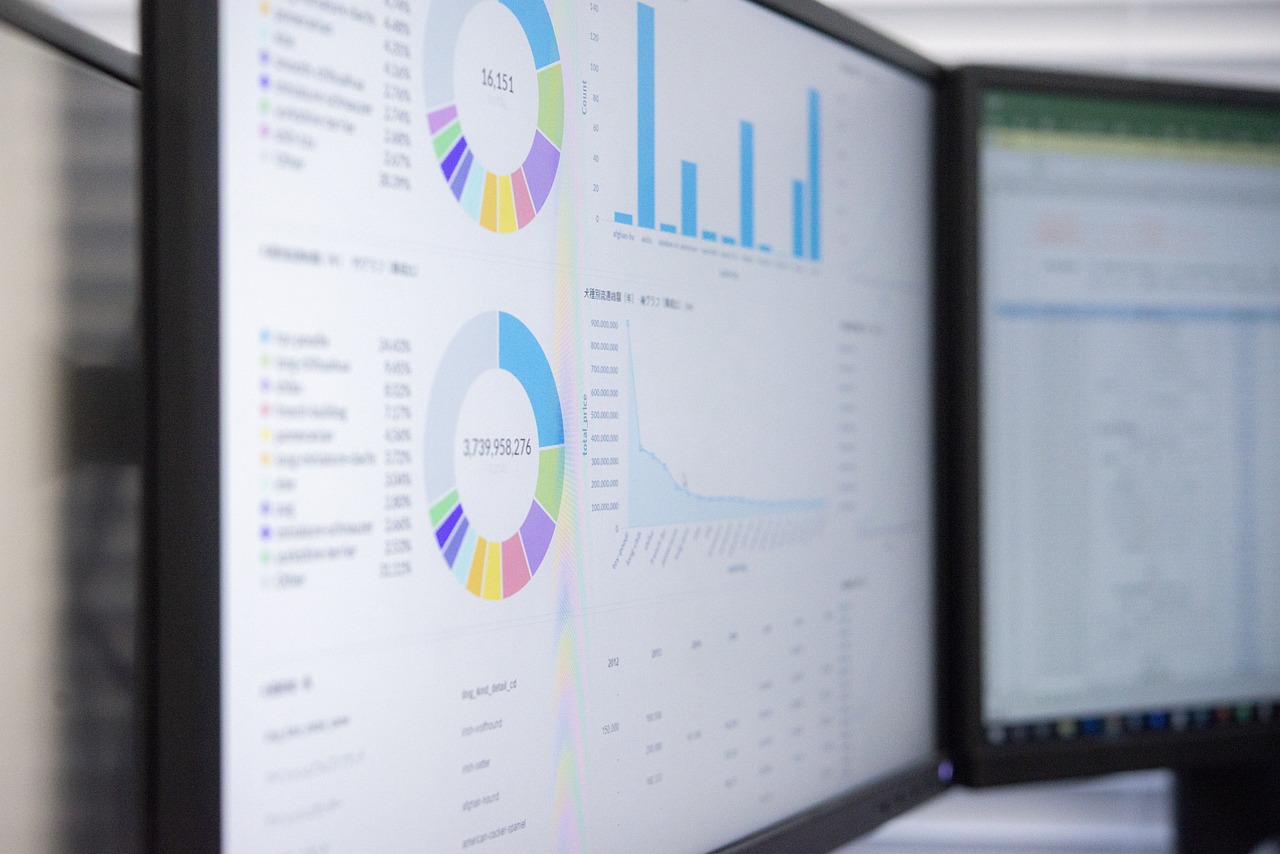
Descriptive Statistics
When diving into the world of forecasting, serve as the compass that guides us through the vast sea of historical data. They provide a clear and concise summary of data sets, allowing forecasters to grasp the underlying patterns and trends at a glance. Think of descriptive statistics as the highlights reel of a sports game—while it doesn’t show every single play, it captures the essence of the action and helps you understand who’s winning and why.
Descriptive statistics primarily include measures such as the mean, median, and standard deviation, each playing a vital role in data interpretation. The mean gives us the average value, offering a central point around which data points cluster. However, it can be skewed by outliers—those pesky data points that don’t quite fit in. This is where the median comes into play, providing a more robust measure of central tendency by representing the middle value in a sorted list of numbers. When you think about it, the median is like the referee in a game, ensuring that the extremes don’t unfairly influence the outcome.
Furthermore, the standard deviation is crucial for understanding data variability. It tells us how spread out the data points are around the mean. A low standard deviation indicates that the data points are close to the mean, while a high standard deviation suggests a wider spread. Imagine it as the difference between a tightly-knit team that plays well together and a team where players are all over the field—both can be effective, but their strategies and outcomes will differ significantly.
To make this concept more tangible, let’s look at a simple table that summarizes these descriptive statistics:
Measure | Description | Example |
---|---|---|
Mean | The average of all data points. | For data points 2, 3, 5, the mean is (2+3+5)/3 3.33 |
Median | The middle value when data points are arranged in order. | For data points 1, 3, 3, 6, 7, the median is 3. |
Standard Deviation | A measure of the amount of variation or dispersion in a set of values. | For data points 1, 2, 3, the standard deviation is 0.82. |
Incorporating descriptive statistics into the forecasting process not only enhances the understanding of historical data but also lays the groundwork for more advanced analyses. By summarizing data effectively, forecasters can identify trends and make informed predictions about future outcomes. It’s like having a well-organized toolbox—when you know where everything is, you can work more efficiently and effectively!
- What are descriptive statistics? Descriptive statistics summarize and describe the main features of a data set, providing a simple overview of its main characteristics.
- Why are descriptive statistics important in forecasting? They allow forecasters to quickly understand data trends and patterns, which is essential for making informed predictions.
- How do I calculate the mean and median? The mean is calculated by adding all data points and dividing by the number of points. The median is found by arranging the data points in order and selecting the middle value.
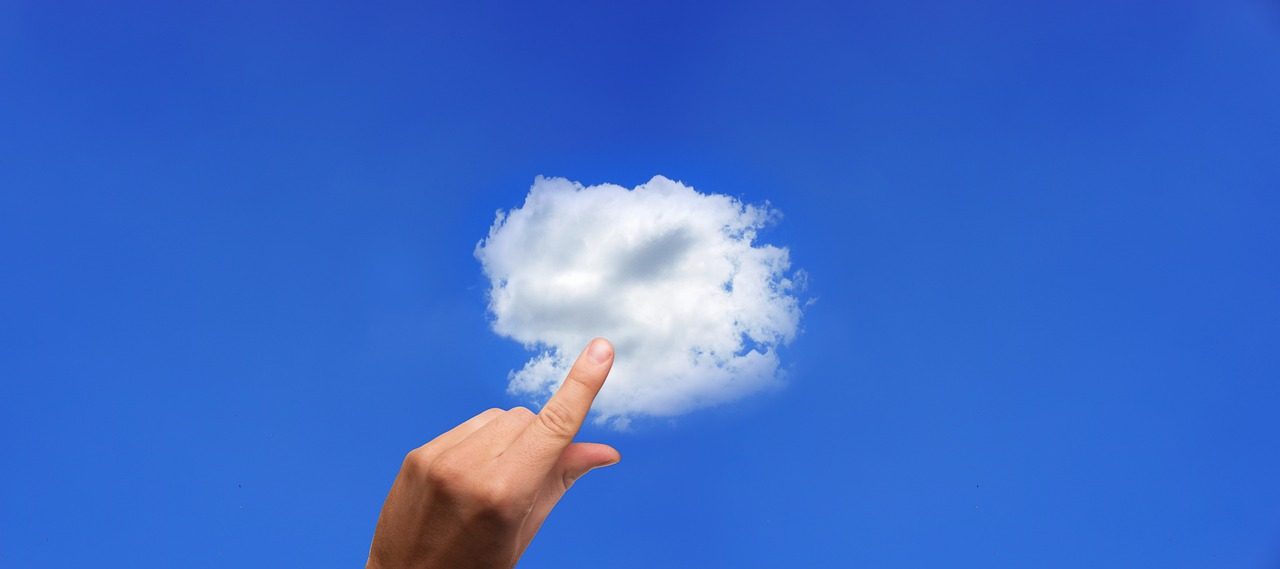
Inferential Statistics
When it comes to making predictions about future trends, plays a pivotal role. Unlike descriptive statistics, which merely summarize historical data, inferential statistics allows us to draw conclusions and make forecasts based on a sample of data. Imagine you're trying to predict the outcome of a large sports tournament based on the performance of a few selected teams. By analyzing their past performances, you can infer how they might perform in future matches, even if you don’t have data on every team.
One of the most compelling aspects of inferential statistics is its ability to account for uncertainty. In the world of forecasting, uncertainty is a constant companion. This method helps us navigate through it by providing a framework for making educated guesses. For example, if you have data from a survey about consumer preferences, inferential statistics enables you to estimate the preferences of the entire population, not just those who participated in the survey. This is achieved through techniques such as confidence intervals and hypothesis testing.
To put it simply, inferential statistics is like a crystal ball for data analysts. It helps in:
- Making Predictions: Using sample data to predict trends for a larger population.
- Testing Hypotheses: Validating assumptions about data through statistical tests.
- Estimating Parameters: Providing estimates of population parameters based on sample statistics.
Let’s consider an example: a company wants to launch a new product and conducts a survey among 100 potential customers. Using inferential statistics, they can analyze the survey results and infer how the entire market might react to the product. This approach not only saves time and resources but also enhances decision-making capabilities.
Moreover, inferential statistics can also help identify significant trends and patterns that may not be immediately obvious. For instance, you might find that a particular demographic shows a strong preference for a product, which could lead to targeted marketing strategies. In essence, it empowers businesses to make data-driven decisions with a higher degree of confidence.
In conclusion, inferential statistics is an essential tool in the forecasting toolkit, bridging the gap between historical data and future predictions. By leveraging sample data to make broader inferences, organizations can navigate uncertainty and make informed decisions that drive success.
- What is the difference between descriptive and inferential statistics?
Descriptive statistics summarizes data, while inferential statistics makes predictions and inferences about a population based on a sample. - How can inferential statistics be applied in business?
It can be used for market research, product testing, and understanding customer preferences to guide strategic decisions. - What are confidence intervals?
Confidence intervals provide a range of values that likely contain the population parameter, offering insight into the reliability of an estimate.
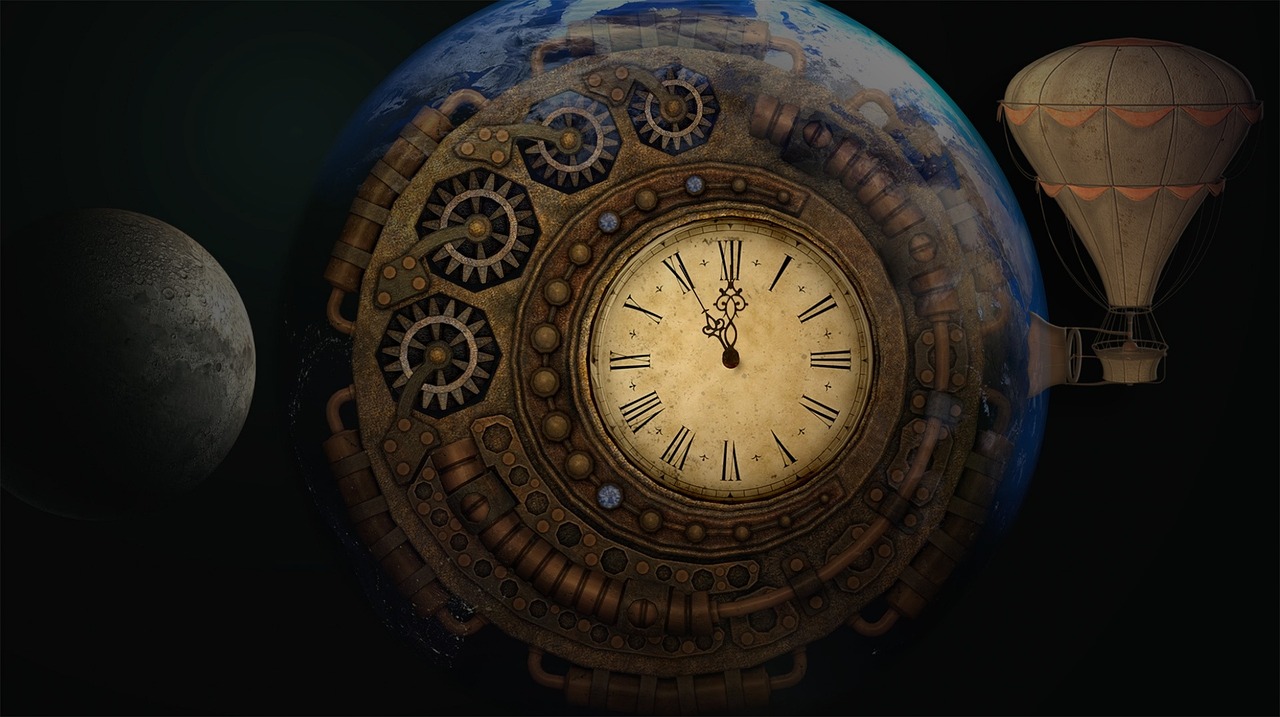
Time Series Analysis
Time series analysis is a powerful statistical technique that focuses on data points collected or recorded at specific time intervals. Imagine you're a detective piecing together clues from a series of events; time series analysis allows you to see how these events unfold over time, revealing hidden patterns that can be crucial for making predictions about the future. This method is particularly valuable because it helps identify seasonal trends, cyclical patterns, and long-term movements in data, which are essential for businesses aiming to stay ahead of the curve.
One of the key aspects of time series analysis is its ability to highlight seasonality. For instance, if you run a retail business, analyzing sales data over several years might reveal that your sales spike every holiday season. This insight can help you plan inventory and marketing strategies effectively. Similarly, cyclical patterns, which occur over longer periods, can indicate broader economic trends that might impact your business. By recognizing these cycles, you can make more informed decisions about when to invest in new projects or when to hold back.
Moreover, time series analysis often employs various techniques to smooth out fluctuations in data, making it easier to identify underlying trends. Techniques such as moving averages and exponential smoothing are commonly used. A moving average, for example, takes the average of a specific number of past data points to provide a clearer picture of the trend. This can be particularly useful when your data is noisy or has a lot of variability.
To illustrate the effectiveness of time series analysis, consider the following example:
Year | Sales ($) |
---|---|
2020 | 50,000 |
2021 | 75,000 |
2022 | 100,000 |
2023 | 120,000 |
In this table, you can see a clear upward trend in sales over the years. By applying time series analysis, a business can forecast future sales, allowing them to prepare for increased demand and adjust their strategies accordingly. For example, if the trend continues, one might predict sales for 2024 to reach approximately $140,000, based on the historical growth rate.
Ultimately, time series analysis is not just about crunching numbers; it’s about telling a story with your data. By understanding how your data behaves over time, you can make proactive decisions that align with your business goals. Whether you're a small startup or a large corporation, leveraging time series analysis can provide you with the insights necessary to navigate the ever-changing landscape of your industry.
- What is the main purpose of time series analysis?
Time series analysis aims to understand past data points to forecast future trends effectively. - How does seasonality affect forecasting?
Seasonality can create predictable patterns in data, which can significantly influence business planning and strategy. - What tools can I use for time series analysis?
Common tools include Excel, R, and Python, which offer various libraries and functions for performing time series analysis.
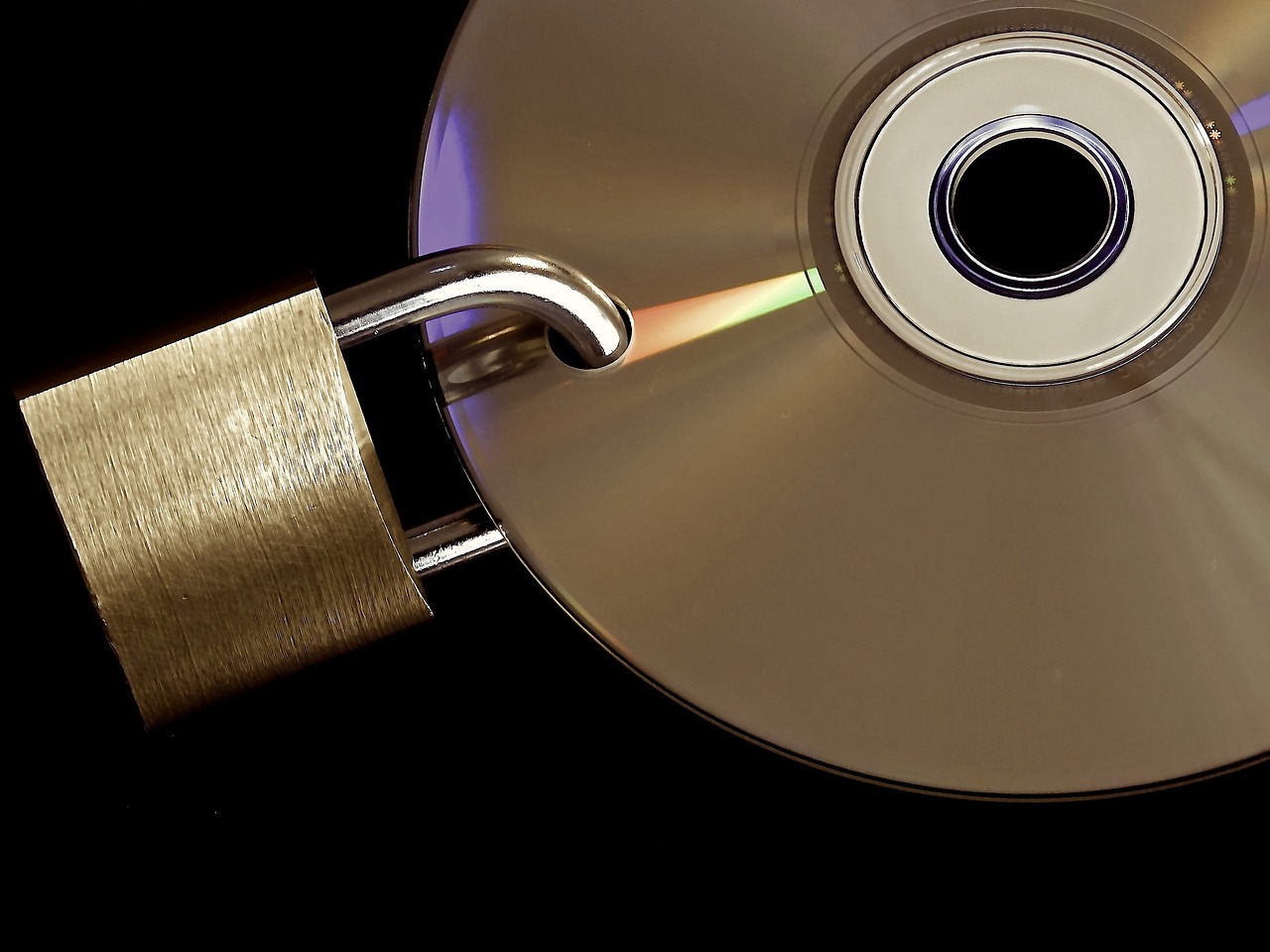
Tools for Forecasting
When it comes to forecasting future trends, having the right tools at your disposal is absolutely crucial. Imagine trying to navigate a ship without a compass; that’s what forecasting is like without the appropriate tools. There are a plethora of options available, ranging from simple tools like spreadsheets to advanced software solutions that leverage cutting-edge technologies. Choosing the right tool can significantly enhance both the accuracy and efficiency of your forecasting efforts.
One of the most widely used tools for forecasting is Microsoft Excel. This ubiquitous software is favored by many businesses due to its accessibility and functionality. Excel offers a variety of built-in functions and templates that can cater to basic forecasting needs. For instance, users can easily create time series graphs, apply moving averages, or even utilize the FORECAST function to make predictions based on historical data. The flexibility of Excel allows users to customize their analyses, which can be particularly beneficial for small to medium-sized enterprises that may not have the budget for more sophisticated tools.
However, as businesses grow and data complexity increases, relying solely on Excel may not be sufficient. This is where advanced forecasting software comes into play. These solutions often provide more robust analytics capabilities, including machine learning algorithms and data visualization tools. For example, platforms like Tableau and IBM Watson Analytics enable organizations to analyze large datasets with ease, uncovering insights that would be nearly impossible to identify using basic tools. Not only do these advanced solutions enhance accuracy, but they also allow for real-time data processing, which is essential in today’s fast-paced business environment.
Moreover, many of these advanced tools come equipped with user-friendly interfaces that make it easier for non-technical users to perform complex analyses. They often include features such as automated forecasting, which can save time and reduce human error. For example, tools like Forecast Pro and SAP Analytics Cloud offer automated forecasting capabilities that can analyze historical data and generate forecasts with minimal input from users, allowing organizations to focus on strategy rather than data crunching.
In summary, the choice of forecasting tools can significantly influence the effectiveness of your forecasting efforts. While Excel is a fantastic starting point for many organizations, advanced software solutions offer capabilities that can take your forecasting to the next level. By leveraging the right tools, businesses can make more informed decisions, ultimately leading to better strategic planning and success in their respective markets.
- What is the best tool for beginners in forecasting?
For beginners, Microsoft Excel is an excellent starting point due to its accessibility and user-friendly features. - Can advanced forecasting tools integrate with existing systems?
Yes, many advanced forecasting tools are designed to integrate seamlessly with existing data management systems, enhancing their functionality. - Is machine learning necessary for effective forecasting?
While machine learning can enhance forecasting accuracy, it is not strictly necessary. Basic statistical methods can still yield valuable insights.
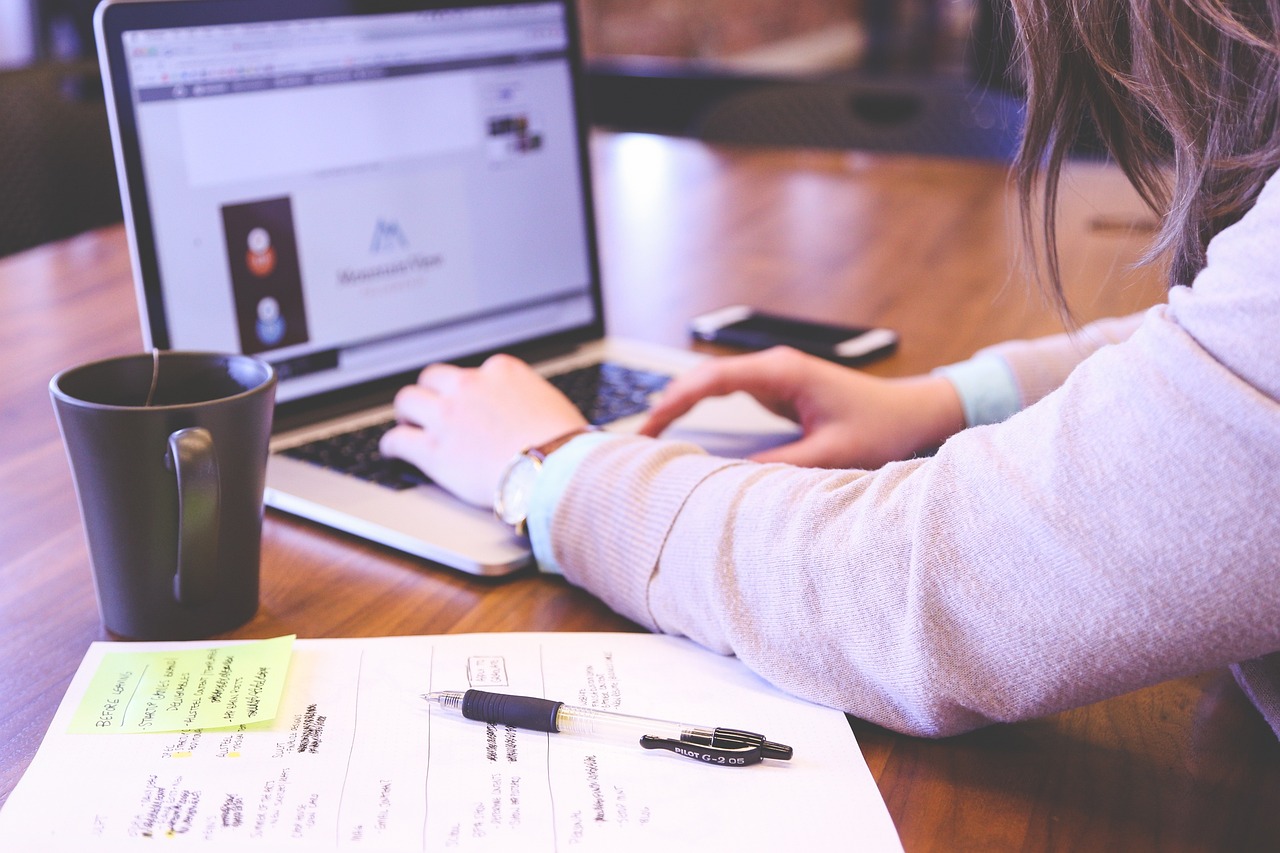
Excel for Forecasting
When it comes to forecasting, Excel stands out as a powerful ally for many businesses, regardless of their size. Its accessibility and user-friendly interface make it a go-to tool for both novices and seasoned analysts. With Excel, you can easily manipulate data, perform calculations, and visualize trends, all in one place. Imagine having a toolbox filled with various instruments, each designed to help you carve out insights from your data—this is precisely what Excel offers!
One of the most compelling features of Excel is its array of built-in functions that cater specifically to forecasting needs. For instance, functions like FORECAST
, TREND
, and LINEST
allow users to project future values based on historical data. The beauty of these functions lies in their simplicity; you don't need to be a data scientist to harness their power. Just input your data, select the appropriate function, and watch as Excel churns out predictions that can guide your business decisions.
Moreover, Excel allows for the creation of visual representations of data through charts and graphs. Visualizing data is crucial because it transforms raw numbers into understandable trends. Consider a line graph that showcases sales figures over the last five years—this visual can reveal patterns that might not be immediately apparent in a table of numbers. By presenting data visually, Excel enables stakeholders to grasp complex information quickly, facilitating more informed discussions and decisions.
However, while Excel is incredibly versatile, it does have its limitations. For instance, handling large datasets can lead to performance issues, and the absence of advanced analytics features may restrict deeper insights. To illustrate, let’s compare Excel with advanced forecasting software in a simple table:
Feature | Excel | Advanced Software |
---|---|---|
Data Handling | Good for small to medium datasets | Optimized for large datasets |
Built-in Functions | Basic forecasting functions | Advanced algorithms and machine learning |
Visualization | Charts and graphs | Dynamic dashboards and interactive visualizations |
Ease of Use | User-friendly for beginners | Steeper learning curve |
In summary, Excel is an excellent starting point for organizations looking to dive into forecasting. Its combination of accessibility, functionality, and visualization capabilities makes it a valuable tool for anyone aiming to leverage historical data for future predictions. However, as your forecasting needs grow more complex, it may be beneficial to explore more advanced software solutions that can provide deeper insights and handle larger datasets.
- Can I use Excel for advanced forecasting? While Excel is great for basic forecasting, for more complex analyses, consider advanced software that utilizes machine learning algorithms.
- What are some common forecasting functions in Excel? Functions like
FORECAST
,TREND
, andLINEST
are widely used for making predictions based on historical data. - Is Excel suitable for large datasets? Excel can handle small to medium datasets effectively, but performance may suffer with larger datasets.
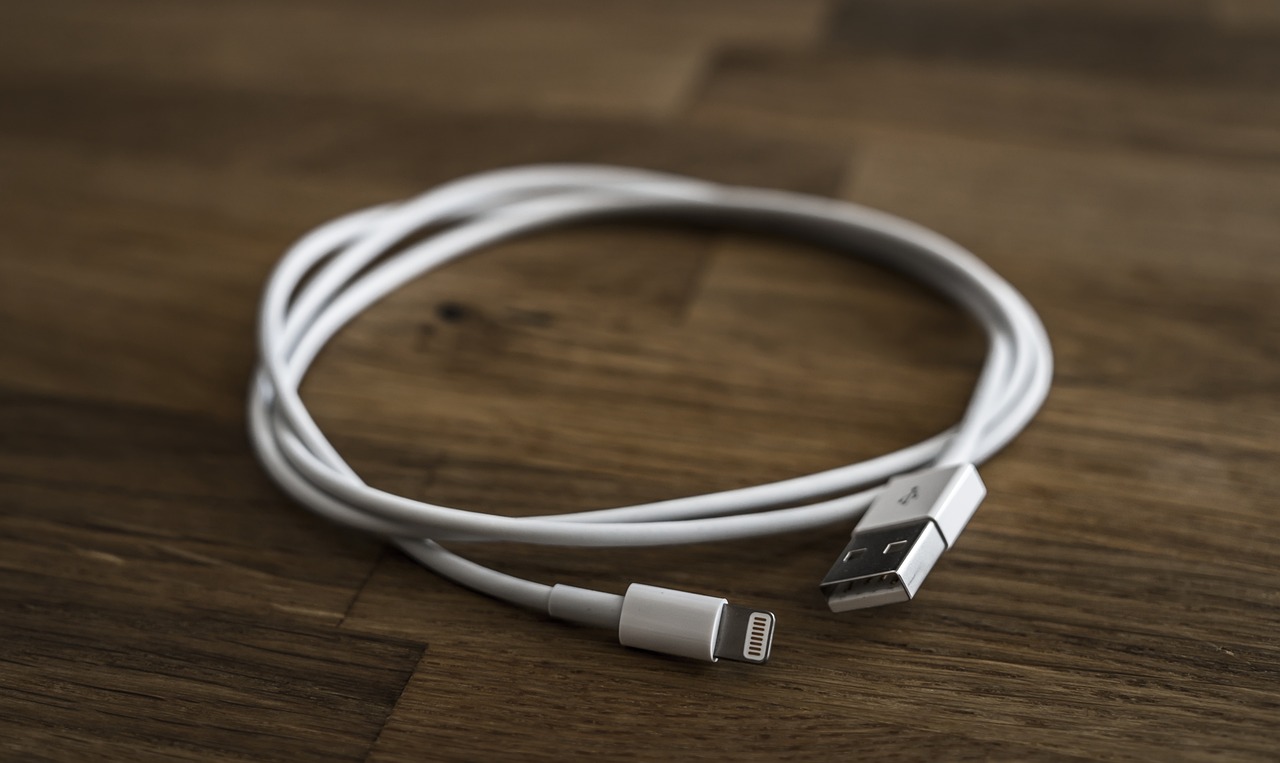
Advanced Software Solutions
In today's fast-paced business environment, relying on basic tools for data analysis can be like trying to catch a fish with your bare hands when you have a perfectly good fishing rod available. have revolutionized the way organizations analyze historical data and forecast future trends. These tools not only enhance accuracy but also provide a level of insight that traditional methods simply cannot match.
One of the standout features of advanced forecasting software is its ability to integrate machine learning algorithms. These algorithms learn from historical data patterns, allowing the software to make predictions that become increasingly accurate over time. Imagine having a personal assistant who learns your preferences and habits; that’s how machine learning enhances forecasting—by adapting to new data and refining its predictions.
Moreover, many advanced software solutions come equipped with data visualization tools. Visual representations of data can make complex information more digestible. For instance, instead of sifting through rows of numbers, you can view trends through interactive graphs and charts. This not only aids in understanding but also facilitates communication with stakeholders who may not be as data-savvy. A well-designed dashboard can transform raw data into compelling stories that drive strategic decisions.
When choosing an advanced software solution, organizations should consider several factors, such as:
- Scalability: Can the software grow with your business needs?
- User-friendliness: Is the interface intuitive enough for all team members?
- Integration capabilities: Does it work well with your existing systems?
- Support and training: What kind of customer support and training resources are available?
In addition to these features, many advanced software solutions offer collaborative tools that enable teams to work together seamlessly. This is particularly important in a world where remote work is becoming the norm. Teams can share insights, compare forecasts, and make collective decisions in real-time, regardless of their physical location. This collaborative aspect not only improves efficiency but also fosters a culture of shared knowledge and accountability.
Ultimately, investing in advanced forecasting software can be a game-changer for organizations looking to harness the power of historical data. By leveraging sophisticated analytics and visualization tools, businesses can not only predict future trends more accurately but also make informed decisions that drive growth and success.
Q1: What are some popular advanced forecasting software solutions?
A1: Some popular advanced forecasting software solutions include Tableau, IBM SPSS, Microsoft Power BI, and Oracle Analytics Cloud. Each of these tools offers unique features tailored to different forecasting needs.
Q2: How does machine learning improve forecasting accuracy?
A2: Machine learning improves forecasting accuracy by analyzing historical data patterns and continuously learning from new data. This allows the software to adjust its predictions based on emerging trends and changes in the data landscape.
Q3: Can small businesses benefit from advanced forecasting software?
A3: Absolutely! Many advanced forecasting tools are scalable and offer solutions tailored for small businesses. These tools can help small businesses make data-driven decisions and stay competitive in their respective markets.
Frequently Asked Questions
- Why is historical data important for forecasting?
Historical data serves as a foundation for making informed predictions about future trends. It provides context, benchmarks, and a clear understanding of past behaviors, which are crucial for effective strategic planning.
- What methods can I use to analyze historical data?
There are several methods for analyzing historical data, including statistical analysis, time series analysis, and regression models. Each method has its own strengths and can be tailored to meet specific forecasting needs.
- How do statistical analysis techniques help in forecasting?
Statistical techniques, like mean, median, and standard deviation, summarize historical data effectively. These techniques enable forecasters to identify trends and anomalies, providing a clearer picture of what to expect in the future.
- What is time series analysis?
Time series analysis involves examining data points collected over specific time intervals. This method helps identify seasonal trends, cyclical patterns, and long-term movements, making it a powerful tool for forecasting.
- What tools are available for forecasting?
There are a variety of tools for forecasting, ranging from simple spreadsheets like Excel to advanced software solutions that utilize machine learning algorithms and data visualization tools. The right choice depends on your specific data needs.
- Is Excel effective for forecasting?
Absolutely! Excel is widely used for forecasting due to its accessibility and extensive functionality. It offers built-in functions and templates that can cater to basic forecasting needs effectively.
- When should I consider advanced forecasting software?
If your organization has complex data needs or requires robust analytics capabilities, advanced forecasting software is the way to go. These solutions provide enhanced features, including machine learning and sophisticated data visualization options.